My 20-year career journey in data science includes working for two of the largest SaaS providers in the market today (Oracle and Salesforce), building the latter’s AI platform (Einstein), and developing other data science products. I have also led evaluation processes for several B2B tech solutions; most notably for risk forecasting tools that support human decision-making.
Today, at Zone7, our team of experts across data science, sports medicine, and performance management is building customized risk forecasting solutions (backed by algorithms and data science) for leading professional sports teams across Europe and North America. We are also ensuring their successful application in each environment through regular communications with its user base of performance specialists (coaches, physios, sports scientists, etc.).
This unique mix of experience across the buy side and sell side of performance tech adoption allows me to develop (and share with you) a curated list of steps that professional sports organizations should follow as they assess the legitimacy and quality of different tech solutions, including AI-based products.
By following these best practices, teams, leagues, and other organizations can run a thorough “truth-seeking” process that allows them to focus on proven and validated solutions and approaches.
1. Demand Validation Studies
While this question may seem obvious, it’s one to ask early on.
All technology service providers should have evidence of their efficacy, especially those providing algorithm-based tech solutions. This is why Zone7 has compiled a mix of named and anonymous client case studies that prove our technology’s positive real-world impact when used appropriately. Our success metrics include reduced injury rates, more value from investments in athletes, increased psychological certainty for performance practitioners, and improved competitive outcomes.
2. Request A Custom Validation Study Using Your Own Datasets and/or Environment
Requesting a custom validation study (after following step 1) tests the solution provider’s ability to:
- Pivot on short notice
- Understand the customer’s specific needs
- Adopt the performance metrics the customer uses to evaluate risk
In the last five years, Zone7 has conducted custom validation studies for several clients across different leagues and sports. We provide them with a retrospective analysis of the client’s historical data from the prior year or season. This exercise verifies our platform’s ability to:
- Identify indicators of heightened injury risk in athletes
- Map these signals to the timeline of actual injury events
- Provide actionable recommendations to potentially reduce injury risk
This process also validates another Zone7 key benefit: our platform is device agnostic and can interpret whatever mix of strength, conditioning, and medical data a sports organization has available.
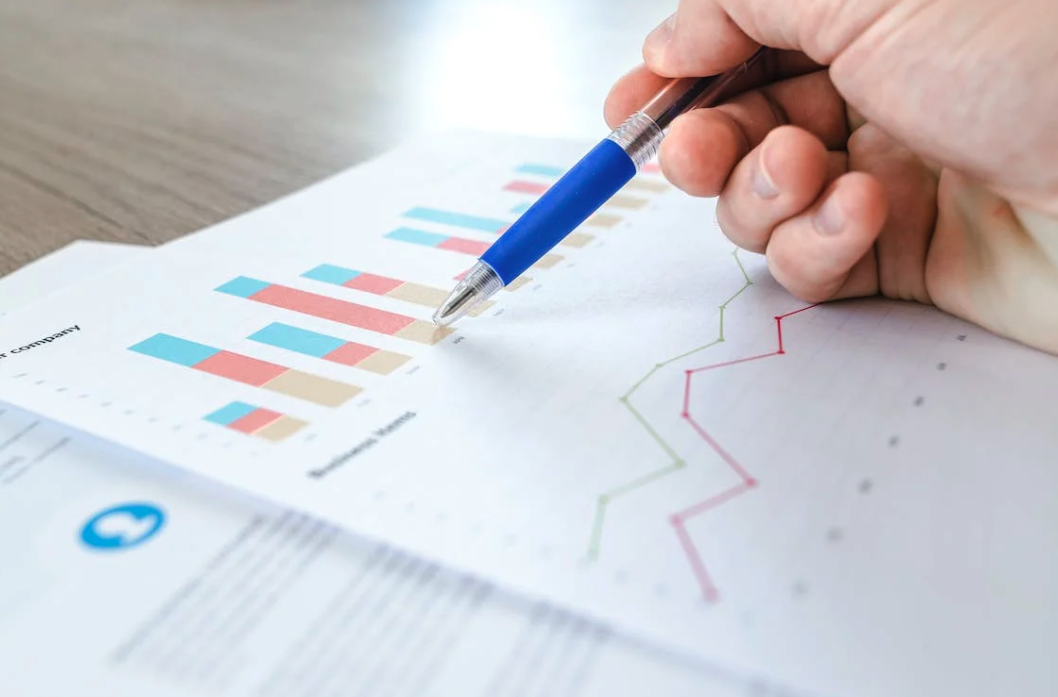
3. Ask To Review A Validation Study’s Underlying Raw Data
Once a vendor performs a custom validation study for your data, they should be willing to provide the study’s raw data, if asked. This request establishes trust with the vendor and verifies that the technology is built on data algorithms, rather than manual analysis. It also allows prospective buyers to verify the KPIs (such as # of alerts or X% reduction in ‘false alarms’) independently.
Having the opportunity to analyze raw data also enables a more educated and comprehensive follow-on discussion with the provider’s multidisciplinary team around key topics like threshold configuration and false positive rates. Some other questions to ask of pre-baked validation studies include:
- What environment does this validation study come from?
- Which sport / team / population of players were analyzed?
- How extensive is the dataset (e.g. the number of risk-related incidents in the study)?
- What are the specific inputs (GPS tracking, strength and conditioning data, risk tolerance level, etc.)?
- Who led the study’s creation and analysis?
- Did other independent parties contribute to the study’s design, execution and validation?
4. Vet The Provider’s Protections Against “Overfitting”
Overfitting refers to cases where an algorithm’s results are skewed to show ‘better’ results. This is typically associated with a (usually unintentional) leakage from the dataset used to TEST an algorithm, which then flows into the dataset used to TRAIN the algorithm.
In other words, the model should “learn” from one set of incidents and be “tested” on a completely separate set of incidents. Otherwise, it will be “overfitted” to successfully detect incidents of one specific type. When a solution provider creates a case study for a specific environment, there should be absolute transparency about whether (and how) any data from this environment was used to inform (aka train) the modeling process.
A good machine learning system avoids overfitting by adhering to strict operational principles of absolute separation between training data and test data. Zone7 always isolates training data and test data through a comprehensive separation policy, which is visible and “transparent” to clients.
Side note: Jo Clubb, a Zone7 ally and sports science expert, has crafted a thorough dictionary of AI terms (such as ‘overfitting’) that readers can leverage as they learn about the intersection of sports science and data science.
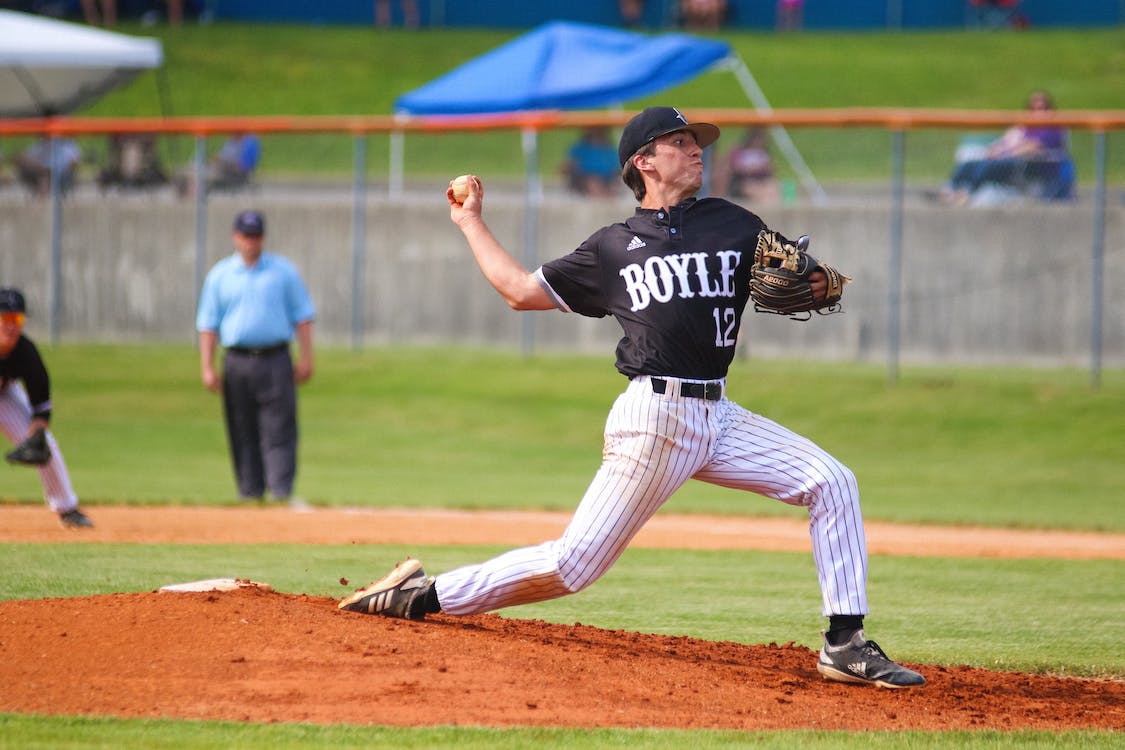
5. Throw A Curve Ball
Unexpected scenarios are the best form of stress testing.
Tasking a solution provider with a separate (yet related) request allows adopters to probe further into a solution’s capabilities and the competence of its engineering team while undergoing due diligence. Curve balls also establish whether an automated solution is truly based on code, or whether its findings are the product of manual analysis disguised as machine-driven.
Zone7 embraces these challenges, which have come in the form of asking our team to recut our model with new data (such as a different combination of internal workload and strength assessment inputs) or requesting a deep dive into past scenarios during a demo session. Operators can see firsthand that we understand the complexities of a real sporting environment.
Final Thoughts
As with any innovative technology that claims to unlock new insights, the data science solutions market has its fair share of credible and less credible offerings. If a solution provider is truly committed to supporting athletes, performance practitioners, and sports organizations, they will not hesitate to meet these requirements.
From our position as a transparent provider, we embrace being tasked with the burden of proof. It is a necessary step that will ultimately foster a stronger, long-term working relationship.
Press your prospective tech solution providers on the legitimacy of their claims, especially those claiming to offer risk forecasting and/or custom algorithms. The potential financial and competitive gains at stake are simply too great.
Here are a handful of other resources to help performance specialists understand, and fully leverage, the full potential of data science and AI for athlete performance management:
– Jo Clubb: Illuminating the Black Box in Search of Greater Transparency in Sports Science
– Jo Clubb: Why Context is a Must in Sports Science AI
– Jo Clubb: Load Monitoring Frequently Asked Questions
Ready to Elevate your Performance? We provide a comprehensive suite of products and services utilizing data and AI to enable greater performance and durability amongst high-performance teams.
To find out more about our work, Book a Demo today.